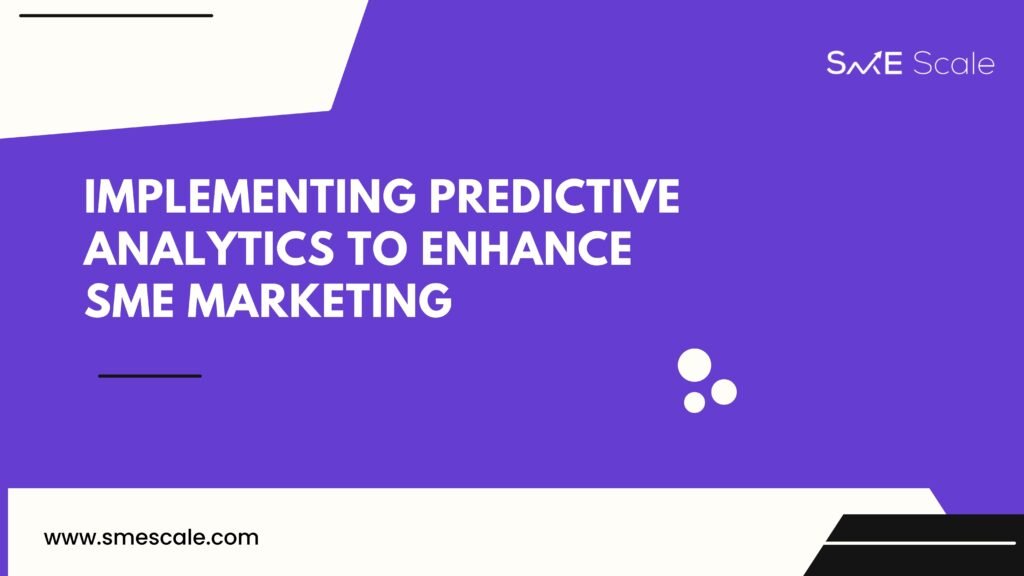
In the increasingly competitive business landscape, small and medium enterprises (SMEs) need to adopt innovative strategies to stay ahead of the curve. One such strategy is predictive analytics, which involves using historical data to forecast future outcomes. For SMEs, predictive analytics can be a game-changer, offering deeper insights into customer behavior, improving marketing effectiveness, and increasing ROI. By leveraging data-driven decision-making, SMEs can not only optimize their marketing efforts but also anticipate customer needs and trends.
This blog will explore how SMEs can implement predictive analytics, highlighting a real-life case study and explaining the psychological principles behind why predictive analytics works so well in marketing.
What is Predictive Analytics?
Predictive analytics refers to the use of data, statistical algorithms, and machine learning techniques to identify the likelihood of future outcomes based on historical data. For SMEs, predictive analytics is particularly useful for:
Customer Behavior Prediction: Understanding which customers are likely to churn, or predicting what products or services they may need next.
Personalization: Tailoring marketing messages based on individual customer behavior.
Campaign Optimization: Forecasting which campaigns will yield the highest returns.
Why Predictive Analytics Matters for SMEs
SMEs often operate with limited marketing budgets, making it essential to maximize the return on investment (ROI) for every campaign. Predictive analytics helps businesses to:
Target the Right Customers: By predicting future behavior, SMEs can focus their marketing efforts on high-value customers, ensuring resources are used efficiently.
Increase Customer Retention: Identifying customers who are likely to churn allows businesses to intervene before losing them.
Boost Revenue: Predictive models help identify upsell and cross-sell opportunities, driving more revenue from existing customers.
Implementing Predictive Analytics: Step-by-Step
1. Data Collection
The first step in implementing predictive analytics is gathering data. SMEs should collect data from multiple sources, such as customer purchase history, website interactions, email engagement, and social media activity. The more comprehensive the data, the better the predictions.
For example:
Purchase Data: Helps in understanding buying patterns.
Behavioral Data: Tracks customer engagement with emails, ads, and other touchpoints.
Demographic Data: Provides insights into customer segmentation and targeting.
2. Data Cleaning and Preparation
Once the data is collected, it must be cleaned and organized. This includes removing duplicates, filling in missing values, and ensuring data consistency. Clean data is crucial for accurate predictions.
3. Choose the Right Predictive Model
There are several predictive models available, depending on the goal:
Regression Analysis: Useful for forecasting continuous outcomes like revenue.
Classification Models: Helpful for predicting categorical outcomes like churn risk.
Clustering Models: Ideal for segmenting customers based on behavior patterns.
4. Implementation and Testing
Once the model is selected, it needs to be implemented and tested. SMEs can use tools like Google Analytics, HubSpot, or IBM Watson to implement predictive analytics models. Testing allows businesses to validate the accuracy of the predictions and make adjustments as needed.
5. Actionable Insights and Optimization
The final step is translating predictions into actionable marketing strategies. For instance, if the model predicts a high churn risk for certain customers, the business can target these customers with personalized offers to retain them.
Real-Life Case Study: Crafty Beverages
Crafty Beverages, a small company that produces and delivers craft beverages, was struggling to retain its subscription-based customers. After initial success, they noticed a drop-off in recurring orders and wanted to improve customer retention while driving more personalized marketing.
The Problem:
Crafty Beverages had a significant number of customers canceling their subscriptions within six months of joining. They needed a way to predict which customers were likely to churn and take proactive steps to retain them.
The Solution:
They decided to implement predictive analytics using customer data from their subscription service. They collected data on purchase frequency, engagement with email campaigns, and delivery locations. Using this data, they developed a churn prediction model to identify customers at risk of canceling their subscriptions.
The Results:
The predictive model successfully identified customers with a high likelihood of churning. Crafty Beverages then created targeted email campaigns offering discounts and personalized product recommendations to these customers. As a result:
They saw a 20% reduction in churn over six months.
Revenue from their subscription service increased by 15%.
The personalized marketing campaigns had a 40% higher engagement rate compared to their previous generic campaigns.
The Psychology Behind Predictive Analytics in Marketing
Predictive analytics taps into several psychological principles that enhance marketing effectiveness:
Personalization and Reciprocity: Personalizing offers based on predictive analytics makes customers feel valued. According to the reciprocity principle, customers are more likely to respond positively when they feel a company is catering to their specific needs.
Anticipation and Loss Aversion: Predicting future behaviors, such as churn, allows businesses to act preemptively. By sending targeted offers before a customer cancels, SMEs can leverage loss aversion, a principle where individuals are more motivated to avoid losing something than to gain something.
Confirmation Bias: By using data to predict a customer’s preferences, businesses can create marketing messages that align with the customer’s expectations. This taps into confirmation bias, where individuals seek information that confirms their existing beliefs or preferences.
Conclusion
For SMEs, predictive analytics is a powerful tool that can enhance marketing efforts by making them more data-driven, personalized, and effective. By predicting customer behavior, businesses can improve retention rates, drive revenue, and maximize ROI. As demonstrated by Crafty Beverages, even small companies can benefit from using predictive analytics to refine their marketing strategies and stay ahead in a competitive market.
By implementing predictive analytics, SMEs can unlock the potential of their customer data, improving both business performance and customer satisfaction.